Data Critique
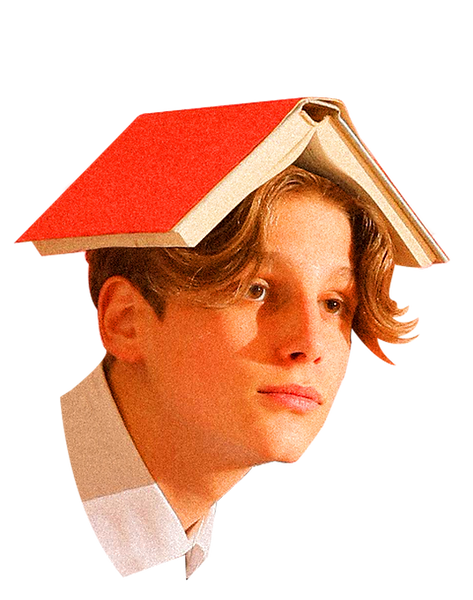
Our CSV file contains information regarding SAT scores and breaks these scores down into two categories: Verbal and Math. Additionally, we are given the states in which students live. Using these three data points, we can analyze the SAT scores of California and West Virginia students. Moreover, we have the income brackets which each state’s students fell in. Using this dataset, we can illustrate possible score advantages that more “well-off” families had over lower-income ones. We also have students’ GPAs, which, coupled with the SAT data, can help us gauge how well students’ GPAs predicted their SAT scores (and the reverse relationship). Overall, our dataset can be used in multiple different ways. We can first look at the states in which students grew up, which may have impacted their SAT scores. Second, we can look at how their family income backgrounds may have impacted their same scores. Third, we can look at how their GPAs may have increased or decreased as a result of their backgrounds. Lastly, when we look at the SAT scores, we can review additional ways they may have been impacted and possibly conduct studies about how different genders performed on the tests.
In our research, our dataset ontology can give us information about the relationship between family income and students’ SAT scores. We looked at both low-income and high-income families across California and West Virginia. This gave us a chance to see the relationships between income and scores. Our income brackets can represent the barriers to education that people with various socioeconomic backgrounds face annually. It can also show evidence for unnoticed biases in academic testing.
While our dataset was extremely expansive, we found several limitations that could potentially play a role in how we interpret the data. First, while our dataset told us about SAT takers’ backgrounds, it did not show us their study habits and the access to proper education that these students had. Second, we did not know how “intelligent” each student was, either through an objective IQ test or a different medium.
Additionally, our dataset lacked circumstantial data; instead, it aggregates information by state and year. In other words, we did not know the environment that each student was living in prior to taking the test, such as their school and their neighborhood. Personal circumstances can severely impact, or improve, a student’s score. If we had a way of getting access to the personal circumstances that surrounded each student, we could get more detailed and better quality data. Lastly, our dataset only mentioned the income of families. An improvement to this would be having other background information, such as their family members’ races, highest levels of education completed, and occupations. The background of their family members will likely have had an impact on the student’s upbringing, wealth, and education.